How can we speed up our learning and make improvements that will help us reduce costs? Typically, people making improvements try to change one thing at a time. The problem with this approach is the complexity of the processes and the interdependent factors we are studying. To be more effective, we need to learn how to test more than one factor at a time. Let’s consider an example.
An improvement team in a hospital system that is supported with citizens’ taxes is attempting to help patients not miss appointments. When someone does not show up for an appointment, this is a loss to the society. The improvement team tried texting the patients one day in advance. This did not seem to make much impact. It was then decided to have a person call the patient personally one day in advance. An improvement advisor happened to overhear this discussion and suggested that the team test two factors at two levels or sometimes referred to as a design which will require 4 runs or tests. Rather than abandon the text idea, test it with the call idea, but add in a lead time factor with a call 1 day and 3 days in advance. The improvement advisor suggested calling three days in advance might allow the patients to plan better. Figure 1 describes the simple designed experiment matrix that was developed. Also included are the percent of no shows as the response variable.
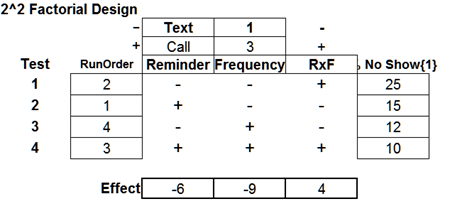
Figure 1: Design Matrix for a Two Factor at Two Level Experiment
From Figure 1, you can see readily that the 4th test that combined the call with 3 days reduced the no show rate to 10%. The next best combination was a text messages at 3 days in advance of the appointment with a 12% no show rate. The team decided to avoid the cost of taking up a person’s time to actually call patients and use the 3 day advance text. One team member suggested setting the computer to also give a 7 day warning. The team agreed to use another test to follow this idea up. The response plots in Figure 2 describes the results from the 4 test runs.
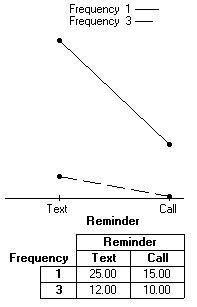
Figure 2: Response Plots for the Factorial design
Before the improvement advisor suggested the designed experiment, the team was ready to run another one factor test: the more expensive idea of adding more people to make personal calls. This would have reduced the no show rate from 25% to 15%, a large improvement but at a significant cost increase. By experimenting with the text and call idea with different frequency levels the team was able to improve while lowering costs.
References:
- Quality Improvement through Planned Experimentation, Ronald Moen, Thomas Nolan, and Lloyd Provost, McGraw-Hill, NY, second edition, 1999